Data sharing in public health emergencies
data "owner" ↔ public interest
... has been problematic in the past
- 2002 Severe acute respiratory syndrome (SARS)
- 2003 H5N1 influenza outbreak. Some countries stopped sharing any data
- 2013-2015 Ebola virus outbreak in West Africa
- 2014-2016 Zika Virus outbreak: Controversies about attribution and reuse
- 2014- H7N9 influenza outbreak: Controversies about attribution and reuse
Different disease -- different scientists and institutions.
Rapid identification of the virus, sequencing, and transparent sharing by Chinese scientists and authorities
- >10 genomes shared on GISAID
- all sequenced cases come from a single source
- diagnostic recommendations shared through WHO
- early days... things are moving fast
Sequences record the spread of pathogens
Mutations accumulate at a rate of $10^{-5}$ per site and day!
images by Trevor Bedford
Frequent mutations imply...
- most viruses in an outbreak/season differ from each other
- transmission chains are can be inferred
- transmission can be ruled out!
- geographic spread can be reconstructed
- age of an outbreak can be estimated
- drug resistance surveillance
- specific mutations might mediate antigenic mismatch
GISRS and GISAID -- Influenza virus surveillance
- comprehensive coverage of the world
- timely sharing of data through GISAID -- often within 2-3 weeks of sampling
- hundreds of sequences per week (in peak months)
→ requires continuous analysis and easy dissemination
→ interpretable and intuitive visualization
Barriers to data sharing: scientists
- Privacy of study participants
- Fear of being scooped/ensure maximal return
- Secondary analysis perceived as freeloading: "data parasites"
- Don't want to be second guessed
- Release and curation is laborious
- Sloppy records
Barriers to data sharing: organizations and governments
- Economic consequences of outbreaks (tourism, agriculture)
- Conflicts between high and low/middle income countries
- Concerns about IP and commercial exploitation
- Legislative/regulatory barriers
Overcoming Barriers
Open vs restricted sharing
- Alternatives to GenBank: GenBank is "public domain", no requirement to credit data producers
- GISAID/EpiFlu: sign-up and agree to terms and conditions
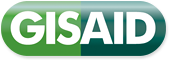
- virological.org: Platform for sharing and discussing molecular epidemiology
- Explicit data reuse terms
- Outline planned projects in white-paper
- Caveat: Very difficult to enforce...
Building Trust
- Peter Bogner coordinated Influenza data sharing
- Andrew Rambaut coordinated Ebola virus data sharing
- During the EBV outbreak, WHO and journals explicitly encouraged data sharing
Make sharing easy and provide incentives!
Acknowledgments
- Trevor Bedford
- Pavel Sagulenko
- James Hadfield
- Emma Hodcroft
- Tom Sibley
- and others