Reconstructing, tracking, and predicting viral spread and evolution
Richard Neher
Biozentrum & SIB, University of Basel
slides at neherlab.org/202205_Cologne.html
Genomic analysis to reconstruct pathogen spread and evolution
Track how pathogens spread: clusters, introductions, etc
Link genotypic and phenotypic changes: immune escape, drug resistance, host adaptation
Human seasonal influenza viruses
slide by Trevor Bedford
Continuous circulation requires steady "supply" of susceptibles
- Birth of immunologically naive individuals
- Waning of pre-existing immunity
- Antigenic change of the virus
Measles (pre vaccine)
- extremely rapid infection of naive children
- long-lived immunity → rare re-infection
Influenza
- frequent reinfection of adults
- combination of waning and antigenic evolution
- Approximate equilibrium between immunity and infections
- Influenza viruses evolve to avoid human immunity
- Vaccines need frequent updates
Hemagglutination Inhibition assays
Slide by Trevor Bedford
Antigenic distance tables
- Long list of distances between sera and viruses
- Tables are sparse, only close by pairs
Slide by Trevor Bedford
Integrating antigenic and molecular evolution
- each branch contributes $d_i$ to antigenic distance
- sparse solution for $d_i$ through $l_1$ regularization
RN et al, PNAS, 2016
Integrating antigenic and molecular evolution -- ferret serology
RN et al, PNAS, 2017
Transition from pandemic to endemic: 2009 A/H1N1
Simple SIR models predict over-shoot and extinction
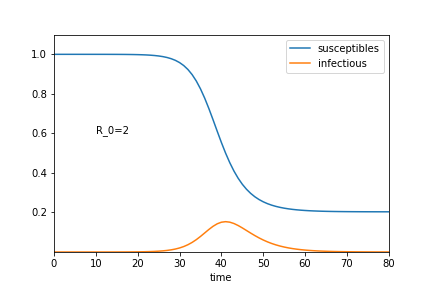
Final susceptibility $S_\infty$: $\log(S_\infty) = - R_0 (S_\infty-1)$
$R_0=2$ → $S_\infty = 0.2$, very small for later $R_0$.
Antigenic evolution during the pandemic-to-endemic transition
Model with strains $a,b,c,\ldots$:
Infections: $ \frac{d}{dt}I_a = \beta S_a I_a - \nu I_a $
Susceptibilty: $ \frac{d}{dt}S_a = -\nu S_a \sum_{b} K_{ab}I_b $
cross-immunity: $K_{ab}$
- early large antigenic steps (relative to cross-immunity range) are necessary to persist
- large cross-immunity range is necessary to prevent speciation
- cross-immunity typically has short and long range components
Yan, RN, Shraiman, eLife, 2019
Current Omicron subvariants of interest
- BA.2 (21L) has essentially taken over.
- BA.4 (22A) and BA.5 (22B) emerged in Southern Africa. Mutations at positions 452 and 486 lead to immune evasion
- BA.2.12.1 (22C) has a mutation at position 452 and is common in the US
nextstrain.org/ncov/gisaid/global
Medium term dynamics of SARS-CoV-2 is very uncertain
- Will we start seeing second and third generation variants, as opposed to sister variants?
- Will we the saltatory dynamics with heavily diverged variants continue?
- Will a more diverse immunity landscape slow down future variant dynamics?
- Will waning/antigenic evolution slow down and give rise to annual or even rarer waves?
____
Influenza and Theory acknowledgments
- Boris Shraiman
- Colin Russell
- Trevor Bedford
- Pierre Barrat
- Oskar Hallatschek
- Le Yan
- All the NICs and WHO CCs that provide influenza sequence data
- The WHO CCs in London and Atlanta for providing titer data
Acknowledgments
Trevor Bedford and his lab -- terrific collaboration since 2014
especially James Hadfield, Emma Hodcroft, Ivan Aksamentov, Cornelius Roemer, Moira Zuber, and John Huddleston
Data we analyze are contributed by scientists from all over the world
Data are shared and curated by GISAID