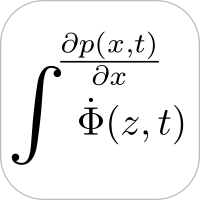
Most of evolutionary theory considers neutral models of evolution, or selection acting on one, two, or maybe a few sites at a time. Most populations, however, are polymorphic at a large number of sites and a fraction of these sites is under selection. Furthermore, fitness might depend on particular combinations of alleles, i.e. alleles might interact and their effect is dependent on the genetic background. The large number of possible genotypes and the constant reshuffling of alleles make multi-locus evolution a challenging subject. Statistical physics, however, provides many examples how complex systems with many degrees of freedom give rise to simple macroscopic behavior. We want to explore how methods from statistical physics can help to develop a general understanding of multi-locus evolution. Our purely theoretical work on properties of rapidly adapting populations has turned out to have surprisingly direct applications: We have used our models and insights to develop methods to predict the evolution of influenza viruses.
Coalescence and genetic diversity in populations under selection
The genetic diversity of a species is shaped by its recent evolutionary history and can be used to infer demographic events or selective sweeps. Most inference methods are based on the null hypothesis that natural selection is a weak evolutionary force. However, many species, particularly pathogens, are under continuous pressure to adapt in response to changing environments. A statistical framework for inference from diversity data of such populations is currently lacking. Toward this goal, we explore the properties of genealogies that emerge from models of continual adaptation. We show that lineages trace back to a small pool of highly fit ancestors, in which simultaneous coalescence of more than two lineages frequently occurs. While such multiple mergers are unlikely under the neutral coalescent, they create a unique genetic footprint in adapting populations. The site frequency spectrum (SFS) of derived neutral alleles, for example, is non-monotonic and has a peak at high frequencies, whereas Tajima's \(D\) becomes more and more negative with increasing sample size. The asymptotics of the SFS can be worked out explicitly. For low frequencies \(x\), we find that the SFS diverges as $$f(x)\sim x^{-2}$$ At high frequency, the SFS increases again and diverges as $$f(x)\sim \frac{1}{(x-1)\log(1-x)}$$ This non-monotonicity is a characteristic feature of multiple merger coalescents.
Since multiple merger coalescents emerge in various evolutionary scenarios characterized by sustained selection pressures, we argue that they should be considered as null-models for adapting populations. Remarkably rather simple scaling arguments map coalescence in sexual populations to results in asexual populations with good accuracy.
Rapid inference of time stamped phylogenies
Mutations that accumulate in the genome of replicating biological organisms can be used to infer their evolutionary history. In case of measurably evolving organisms genomes often reveal their detailed spatio-temporal spread. Such phylodynamic analyses are particularly useful to understand the epidemiology of rapidly evolving viral pathogens. The volume of genome sequences available for different pathogens, however, have increased dramatically over the last couple of years and traditional methods for phylodynamic analysis scale poorly with growing data sets. We have developed TreeTime, a python based framework for phylodynamic analysis using an approximate Maximum Likelihood approach. TreeTime can estimate ancestral states, infer evolution models, reroot trees to maximize temporal signals, estimate molecular clock phylogenies and population size histories. The run time of TreeTime scales linearly with data set size. TreeTime is available as open source package on github and as a web server at treetime.ch.
Publications
2019
Le Yan, Richard A Neher and Boris I Shraiman
eLife, 8 e44205. 10.7554/eLife.44205 pdf bibtex
2018
Richard A. Neher
bioRxiv -- won't submit, 408005. 10.1101/408005 pdf bibtex
2014
Christian Rodelsperger, Richard A. Neher, Andreas M. Weller
Genetics, 196 1153--1165. 10.1534/genetics.113.159855 pdf bibtex
2014
Benjamin H. Good, Aleksandra M. Walczak, Richard A. Neher and Michael M. Desai
PLoS genetics, 10 e1004222. 10.1371/journal.pgen.1004222 pdf bibtex
2013
Richard A. Neher
Annual Review of Ecology, Evolution, and Systematics, 44 195--215. 10.1146/annurev-ecolsys-110512-135920 bibtex
2013
Richard A. Neher, Taylor A. Kessinger and Boris I. Shraiman
Proceedings of the National Academy of Sciences of the United States of America, 110 15836--15841. 10.1073/pnas.1309697110 pdf bibtex
2013
Richard A. Neher, Marija Vucelja, Mark Mezard and Boris I. Shraiman
Journal of Statistical Mechanics: Theory and Experiment, 2013 P01008. 10.1088/1742-5468/2013/01/P01008 pdf bibtex
2013
Richard A. Neher and Oskar Hallatschek
Proceedings of the National Academy of Sciences of the United States of America, 110 437--442. 10.1073/pnas.1213113110 pdf bibtex
2012
Sidhartha Goyal, Daniel J. Balick, Elizabeth R. Jerison
Genetics, 191 1309--1319. 10.1534/genetics.112.141291 bibtex
2012
Philipp W. Messer and Richard A. Neher
Genetics, 191 593--605. 10.1534/genetics.112.138461 bibtex
2011
R. A. Neher and B. I. Shraiman
Genetics, 188 975--996. 10.1534/genetics.111.128876 pdf bibtex
2010
R. A. Neher, B. I. Shraiman and D. S. Fisher
Genetics, 184 467--481. 10.1534/genetics.109.109009 pdf bibtex
2009
Richard A. Neher and Boris I. Shraiman
Proceedings of the National Academy of Sciences of the United States of America, 106 6866--6871. 10.1073/pnas.0812560106 pdf bibtex