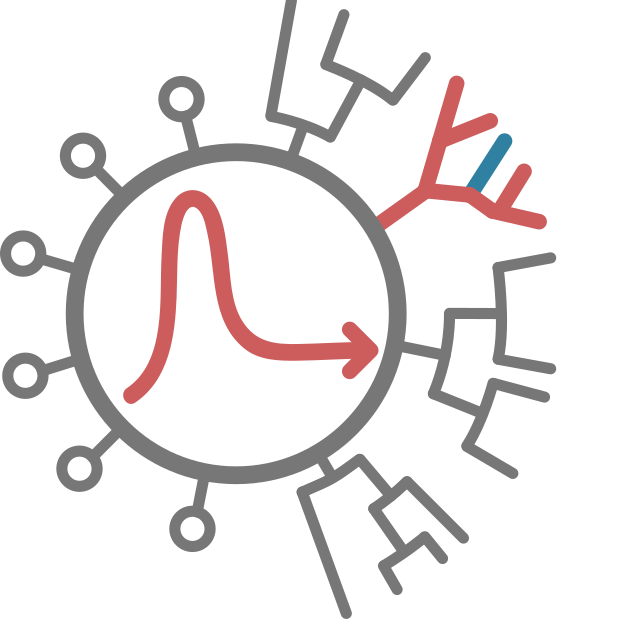
related posts
HIV has a high mutation rate, a generation time of about two days, and different viruses within a patient recombine their genetic material frequently. This combination of factors allows HIV to evolve very rapidly and evade the human immune system to establish a persistent infection. If treated with antiviral drugs, resistance can emerge rapidly unless a combination therapy is used. The genetic diversity of the viral population within a single patient reaches a few percent in certain parts of the HIV genome, which exceeds the divergence between human and chimp. Understanding the evolution of the virus is central to developing vaccines and to minimize drug resistance. In addition, HIV provides an ideal model system in which fundamental questions of evolution can be addressed. In contrast to most other model systems of evolution, time series data is available for HIV evolution which is much more informative about the evolutionary process than the snap shot typical of less rapidly evolving populations.
Whole-genome deep sequencing of HIV-1
We performed whole-genome deep sequencing of HIV-1 populations in 9 untreated patients, with 6-12 longitudinal samples per patient spanning 5-8 years of infection. This work was led by Fabio and is the product of a fantastic collaboration with the group of Jan Albert at the Karolinska Institute in Stockholm. We show that one third of common mutations are reversions towards the ancestral HIV-1 sequence which occur throughout infection with a rate that increases with conservation. Patterns of minor diversity are reproducible between patients and mirror global HIV-1 diversity, suggesting a universal landscape of fitness costs that control diversity. Frequent recombination limits linkage disequilibrium to about 100bp. However, hitch-hiking due to the remaining short range linkage causes levels of synonymous diversity to be inversely related to the speed of evolution.
All data we generated as part of this project are available at hiv.biozentrum.unibas.ch.
Mutation rates and fitness landscapes
In total, we sequenced almost 100 viral population at great depth. From those data, we can determine how rare mutations accumulate over time within infected individuals. We used this mutation accumulation to estimate the mutation rate of HIV in vivo: We estimate that the rate at which nucleotides mutate is about $10^{-5}$ per site and day. If mutations are detrimental to virus replication, the frequency of these variants will stay low despite a constant influx of new mutations. We have used this balance between mutation and selection to estimate the selection coefficient at each site in the genome.
Latent reservoir
HIV not only evolves fast, but also integrates into the genome of human T-cells as latent provirus. Latent virus is the principal barrier to cure because latent virus reseeds an active HIV infection is therapy is stopped. It is therefore critical to understand how this latent reservoir is maintained. In particular, whether the virus constantly replicates in parts of the body with insufficient drug penetration or whether the virus survives in long-lived T-cell lineages is hotly debated.
We studied the evolution of HIV before and after therapy in great detail and
find no evidence of consistent viral evolution after the start of therapy.
To the contrary, virus isolated from the genome of human cells is an almost
perfect snapshot of the virus that circulated right before therapy was initiated.
The graph below shows that evolutionary distance the virus population diverged over
time before and after therapy. Once therapy is started, there is no evidence of
further evolution.
Latently integrated HIV remains very similar to virus circulating before start of treatment.
Publications
2022
Valentin Druelle and Richard A Neher
Virus Evolution, 9 veac118. 10.1093/ve/veac118 bibtex
2018
Laurelle Jackson, Jessica Hunter, Sandile Cele
eLife, 7 e30134. 10.7554/eLife.30134 pdf bibtex
2017
Vadim Puller, Richard Neher and Jan Albert
PLOS Computational Biology, 13 e1005775. 10.1371/journal.pcbi.1005775 pdf bibtex
2017
Fabio Zanini, Vadim Puller, Johanna Brodin, Jan Albert and Richard A. Neher
Virus Evol, 3 10.1093/ve/vex003 pdf bibtex
2016
Fabio Zanini, Johanna Brodin, Jan Albert and Richard A. Neher
Virus research, 10.1016/j.virusres.2016.12.009 bibtex
2016
Johanna Brodin, Fabio Zanini, Lina Thebo
eLife, 5 10.7554/eLife.18889 pdf bibtex
2016
Mikael Boulle, Thorsten G. Muller, Sabrina Dahling
PLoS pathogens, 12 e1005964. 10.1371/journal.ppat.1005964 pdf bibtex
2015
Fabio Zanini, Johanna Brodin, Lina Thebo
eLife, 4 10.7554/eLife.11282 pdf bibtex
2015
Johanna Brodin, Charlotte Hedskog, Alexander Heddini
PloS one, 10 e0119123. 10.1371/journal.pone.0119123 pdf bibtex
2013
Taylor A. Kessinger, Alan S. Perelson and Richard A. Neher
Frontiers in immunology, 4 252. 10.3389/fimmu.2013.00252 pdf bibtex
2013
Fabio Zanini and Richard A. Neher
Journal of virology, 87 11843--11850. 10.1128/JVI.01529-13 pdf bibtex
2013
Vitaly V. Ganusov, Richard A. Neher and Alan S. Perelson
Journal of statistical mechanics (Online), 2013 P01010. 10.1088/1742-5468/2013/01/P01010 pdf bibtex
2010
Richard A. Neher and Thomas Leitner
PLoS computational biology, 6 e1000660. 10.1371/journal.pcbi.1000660 pdf bibtex